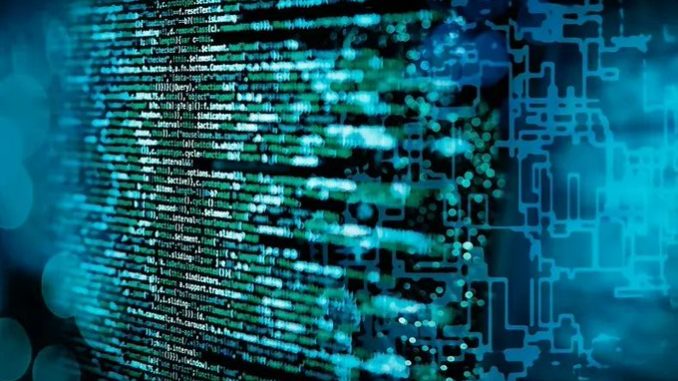
AI models for health care that predict disease are not as accurate as reports might suggest. Here’s why
We use tools that rely on artificial intelligence (AI) every day, with voice assistants like Alexa and Siri being among the most common. These consumer products work reasonably well—Siri understands most of what we say—but they are by no means perfect. We accept their limitations and adapt how we use them until they get the right answer, or we give up. After all, the consequences of Siri or Alexa misunderstanding a user request are usually minor.
However, mistakes by AI models that support doctors’ clinical decisions can mean life or death. Therefore, it’s critical that we understand how well these models work before deploying them. Published reports of this technology currently paint a too-optimistic picture of its accuracy, which at times translates to sensationalized stories in the press. Media are rife with discussions of algorithms that can diagnose early Alzheimer’s disease with up to 74 percent accuracy or that are more accurate than clinicians. The scientific papers detailing such advances may become foundations for new companies, new investments and lines of research, and large-scale implementations in hospital systems. In most cases, the technology is not ready for deployment.
Here’s why: As researchers feed data into AI models, the models are expected to become more accurate, or at least not get worse. However, our work and the work of others has identified the opposite, where the reported accuracy in published models decreases with increasing data set size.
The cause of this counterintuitive scenario lies in how the reported accuracy of a model is estimated and reported by scientists. Under best practices, researchers train their AI model on a portion of their data set, holding the rest in a “lockbox.” They then use that “held-out” data to test their model for accuracy. For example, say an AI program is being developed to distinguish people with dementia from people without it by analyzing how they speak. The model is developed using training data that consist of spoken language samples and dementia diagnosis labels, to predict whether a person has dementia from their speech. It is then tested against held-out data of the same type to estimate how accurately it will perform. That estimate of accuracy then gets reported in academic publications; the higher the accuracy on the held-out data, the better the scientists say the algorithm performs.