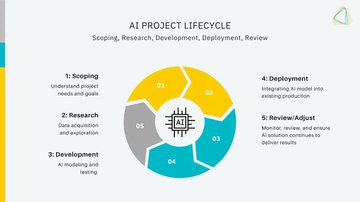
When I teach my AI and business workshop at Stanford, one of the most surprising and insightful topics is the lifecycle of an AI project. Many assume it’s just another software project (far from it!), and they are completely caught off guard when they realize the difference in pace, costs, and data emphasis.
An AI project is a big deal. It marks the high point of an organization’s transition into an AI-driven business, so it should come as no surprise when it impacts all aspects of the company. AI projects are multidisciplinary and often massive undertakings, requiring a competent development team and effective business leaders.
While an AI project never truly “ends” due to the need for adjustments and refinements along the way, there is a point when the product can be launched. This “deployment” stage doesn’t coincide with the end of the project, but rather with the model readiness/maturity stage. This final product can help an organization dramatically increase its value and position within its industry.
With that said, it’s not an easy task arriving at that end product. If you want to minimize any risks and set your business up for the best chance of success, it’s essential to understand each phase of an AI project’s lifecycle.
It can be broken down into two stages, each having two phases.
- Pilot Stage: Includes the scoping and research phases.
- Implementation Stage: Includes the development and deployment phases.
Let’s break down each one of these stages and phases, what they mean, and how they lead to a successful AI project.