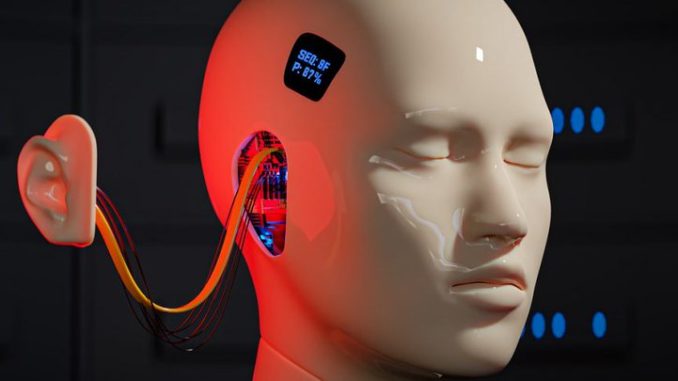
Based on a recently published NCC (National Computing Center) whitepaper, the growing number of organizations creating and deploying machine learning solutions is highly concerning. The aforementioned whitepaper provides a list of attack types which might be carried out against machine learning systems.
According to an InfoQ overview, machine learning systems are subject to specific forms of attacks in addition to more traditional attacks that may attempt to exploit infrastructure or applications bugs, or other kinds of issues.
The first risk that machine learning models face is that they contain code that is executed when the model is loaded or when a particular condition is met. This means an attacker may craft a model containing malicious code and have it executed for a variety of purposes, including leaking sensitive information, installing malware, producing output errors, and so on.
Downloaded models should be treated in the same way as downloaded code; the supply chain should be verified, the content should be cryptographically signed, and the models should be scanned for malware if possible. The NCC Group claims to have successfully exploited this kind of vulnerability.
An additional type of attack that might come into play is adversarial perturbation attacks, where an attacker may craft an input that causes the machine learning system to return results of their choice. This approach could be used to tamper with authentication systems, content filters, and so on.
Membership inference attacks threaten this field too. These attacks are able to expose if an input was part of the model training set. Model inversion attacks allow attackers to gather sensitive data in the training set; data poisoning backdoor attacks consist in inserting specific items into the training data of a system to cause it to respond in some predefined way.