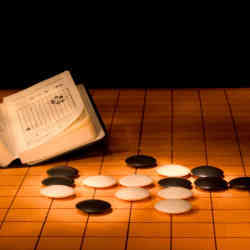
In the world of deep-learning artificial intelligence (AI), the ancient board game Go looms large. Until 2016, the best human Go player could still defeat the strongest Go-playing AI. That changed with DeepMind’s AlphaGo, which used deep-learning neural networks to teach itself the game at a level humans cannot match. More recently, KataGo has become popular as an open source Go-playing AI that can beat top-ranking human Go players.
Last week, a group of AI researchers published a paper outlining a method to defeat KataGo by using adversarial techniques that take advantage of KataGo’s blind spots. By playing unexpected moves outside of KataGo’s training set, a much weaker adversarial Go-playing program (that amateur humans can defeat) can trick KataGo into losing.
To wrap our minds around this achievement and its implications, we spoke to one of the paper’s co-authors, Adam Gleave, a Ph.D. candidate at UC Berkeley. Gleave (along with co-authors Tony Wang, Nora Belrose, Tom Tseng, Joseph Miller, Michael D. Dennis, Yawen Duan, Viktor Pogrebniak, Sergey Levine, and Stuart Russell) developed what AI researchers call an « adversarial policy. » In this case, the researchers’ policy uses a mixture of a neural network and a tree-search method (called Monte-Carlo Tree Search) to find Go moves.