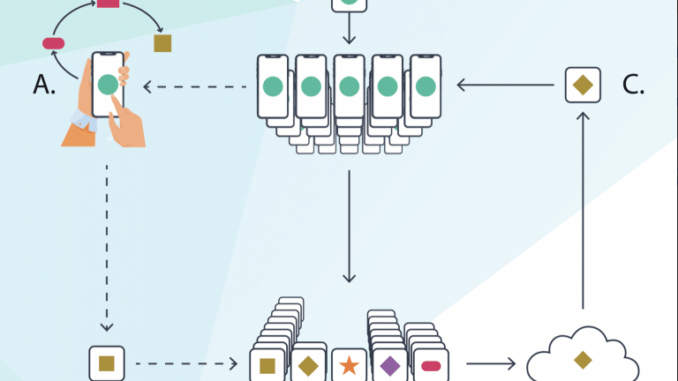
Large volumes of data are required for training machine learning models. The trained model is run on a cloud server that users can access through various applications such as web search, translation, text production, and picture processing, which is the standard procedure for establishing machine learning applications. The application must transfer the user’s data to the server where the machine learning model is stored every time it wishes to use it, creating privacy, security, and processing issues.
Fortunately, developments in edge AI have allowed sensitive user data to be avoided from being sent to application servers. This current area of study, also known as TinyML, aims to construct machine learning models that fit smartphones and other consumer devices, making on-device inference possible. Even if the device is not connected to the internet, these applications can continue functioning. The on-device inference is more energy-efficient in many applications than transferring data to the cloud.