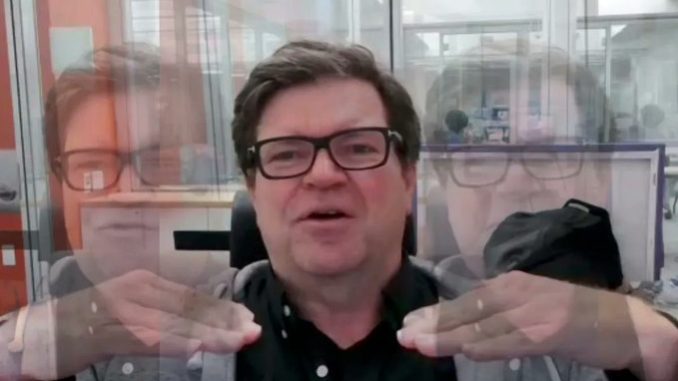
So-called energy-based models, which borrow concepts from statistical physics, may lead the way to ‘abstract prediction,’ says Yann LeCun, allowing for a ‘unified world model’ for AI capable of planning.
Three decades ago, Yann LeCun, while at Bell Labs, formalized an approach to machine learning called convolutional neural networks that would prove to be profoundly productive in solving tasks such as image recognition. CNNs, as they’re commonly known, are a workhorse of AI’s deep learning, winning LeCun the prestigious ACM Turing Award, the equivalent of a Nobel for computing, in 2019.
These days, LeCun, who is both a professor at NYU and chief scientist at Meta, is the most excited he’s been in 30 years, he told ZDNet in an interview last week. The reason: New discoveries are rejuvenating a long line of inquiry that could turn out to be as productive in AI as CNNs are.
That new frontier that LeCun is exploring is known as energy-based models. Whereas a probability function is « a description of how likely a random variable or set of random variables is to take on each of its possible states » (see Deep Learning, by Ian Goodfellow, Yoshua Bengio & Aaron Courville, 2019), energy-based models simplify the accordance between two variables. Borrowing language from statistical physics, energy-based models posit that the energy between two variables rises if they’re incompatible and falls the more they are in accord. This can remove the complexity that arises in « normalizing » a probability distribution.