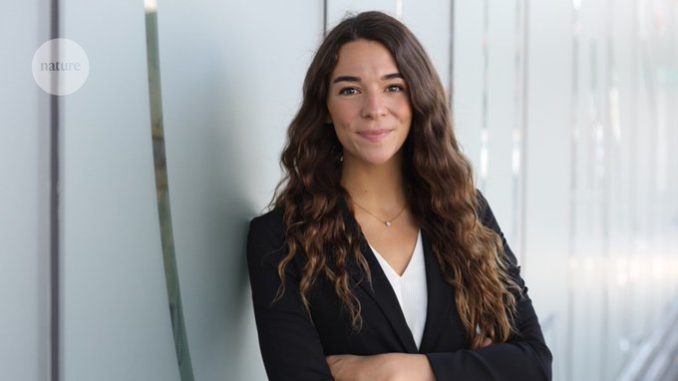
Anna Severin explains how her team used machine learning to try to assess the quality of thousands of reviewers’ reports.
Do more-highly cited journals have higher-quality peer review? Reviews are generally confidential and the definition of ‘quality’ is elusive, so this is a difficult question to answer. But researchers who used machine learning to study 10,000 peer-review reports in biomedical journals have tried. They invented proxy measures for quality, which they term thoroughness and helpfulness.
Their work, reported in a preprint article1 in July, found that reviews at journals with higher impact factors seem to spend more time discussing a paper’s methods but less time on suggesting improvements than do reviews at lower-impact journals. However, the differences between high- and low-impact journals were modest and variability was high. The authors say this suggests that a journal’s impact factor is “a bad predictor for the quality of review of an individual manuscript”.
Anna Severin, who led the study as part of her PhD in science policy and scholarly publishing at the University of Bern and the Swiss National Science Foundation (SNSF), spoke to Nature about this work and other efforts to study peer review on a large scale. Severin is now a health consultant at management consultancy Capgemini Invent in Germany.