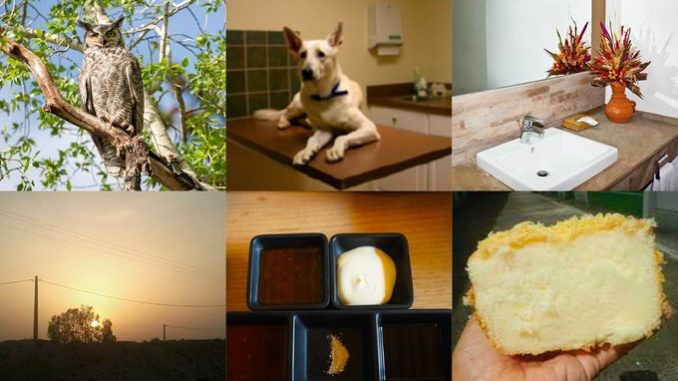
If someone showed you a photo of a crocodile and asked whether it was a bird, you might laugh—and then, if you were patient and kind, help them identify the animal. Such real-world, and sometimes dumb, interactions may be key to helping artificial intelligence learn, according to a new study in which the strategy dramatically improved an AI’s accuracy at interpreting novel images. The approach could help AI researchers more quickly design programs that do everything from diagnose disease to direct robots or other devices around homes on their own.
“It’s supercool work,” says Natasha Jaques, a computer scientist at Google who studies machine learning but who was not involved with the research.
Many AI systems become smarter by relying on a brute-force method called machine learning: They find patterns in data to, say, figure out what a chair looks like after analyzing thousands of pictures of furniture. But even huge data sets have gaps. Sure, that object in an image is labeled a chair—but what is it made of? And can you sit on it?
To help AIs expand their understanding of the world, researchers are now trying to develop a way for computer programs to both locate gaps in their knowledge and figure out how to ask strangers to fill them—a bit like a child asks a parent why the sky is blue. The ultimate aim in the new study was an AI that could correctly answer a variety of questions about images it has not seen before.
Previous work on “active learning,” in which AI assesses its own ignorance and requests more information, has often required researchers to pay online workers to provide such information. That approach doesn’t scale.
So in the new study, researchers at Stanford University led by Ranjay Krishna, now at the University of Washington, Seattle, trained a machine-leaning system not only to spot gaps in its knowledge but to compose (often dumb) questions about images that strangers would patiently answer. (Q: “What is the shape of the sink?” A: “It’s a square.”)
Read more