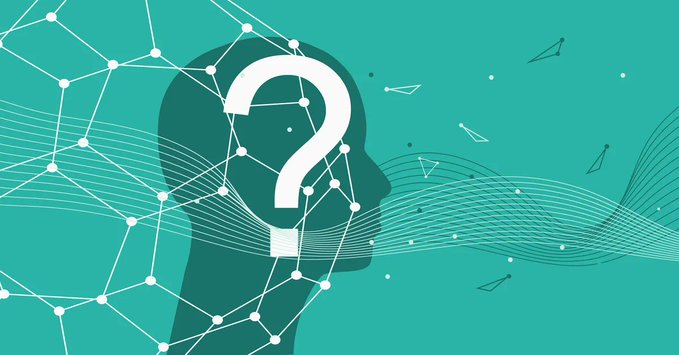
Creating successful artificial intelligence programs doesn’t end with building the right AI system. These programs also need to be integrated into an organization, and stakeholders — particularly employees and customers — need to trust that the AI program is accurate and trustworthy.
This is the case for building enterprisewide artificial intelligence explainability, according to a new research briefing by Ida Someh, Barbara Wixom, and Cynthia Beath of the MIT Center for Information Systems Research. The researchers define artificial intelligence explainability as “the ability to manage AI initiatives in ways that ensure models are value-generating, compliant, representative, and reliable.”
The researchers identified four characteristics of artificial intelligence programs that can make it hard for stakeholders to trust them, and ways they can be overcome:
1. Unproven value. Because artificial intelligence is still relatively new, there isn’t an extensive list of proven use cases. Leaders are often uncertain if and how their company will see returns from AI programs.
To address this, companies need to create value formulation practices, which help people substantiate how AI can be a good investment in ways that are appealing to a variety of stakeholders.
2. Model opacity. Artificial intelligence relies on complex math and statistics, so it can be hard to tell if a model is producing accurate results and is compliant and ethical.
To address this, companies should develop decision tracing practices, which help artificial intelligence teams unravel the mathematics and computations behind models and convey how they work to the people who use them. These practices can include using visuals like diagrams and charts.