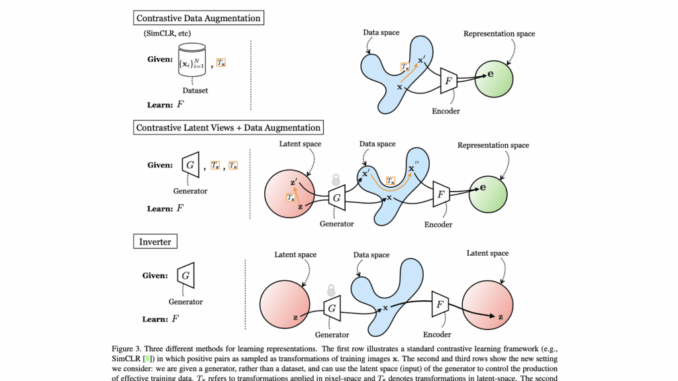
Many machine learning tasks require high-quality data, such as assessing damage in a satellite that negatively affects a model’s performance. Datasets can cost millions of dollars to create if useable data exists, and even the best datasets sometimes contain biases that negatively impact a model’s performance.
Many scientists have been working to answer an intriguing question working with synthetic data sampled from a generative model instead of real data. A generative model is a machine-learning model that requires significantly less memory to keep or share than a dataset. The range and quality of generative models have improved dramatically in recent years.
Synthetic data has the ability to get around some of the privacy and usage rights problems that limit how actual data may be distributed. A generative model could potentially be updated to eliminate particular attributes, such as race or gender, to overcome biases in traditional datasets.
New research by MIT Team develops a method for training a machine learning (ML) model that, rather than requiring a dataset, employs a particular form of ML model to generate exceptionally realistic synthetic data that can train another model for downstream vision tasks.
Their findings suggest that a contrastive representation learning model trained solely on synthetic data may develop visual representations comparable to, if not superior to, those learned from actual data.
After a generative model has been trained on actual data, it can generate synthetic data that is indistinguishable from the original. The training method gives the generative model millions of photos containing objects in a specific class (such as vehicles or cats), after which it learns how to generate comparable objects.