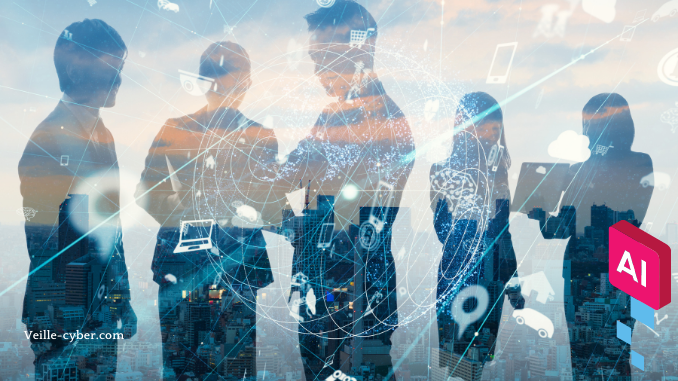
“Perhaps expectations are too high, and… this will eventually result in disaster. Suppose that five years from now, funding collapses miserably as autonomous vehicles fail to roll. Every startup company fails. And there’s a big backlash so that you can’t get money for anything connected with AI. Everybody hurriedly changes the names of their research projects to something else. This condition is called the AI Winter,” said AI expert Drew McDermott in 1984.
In her latest paper titled ‘Why AI is Harder Than We Think’, AI researcher and ousted Google employee Melanie Mitchell, explained how research in AI often follows a cyclic pattern: periods of rapid progress, successful commercialisation, heavy public and private investments, called AI Spring, is often followed by AI winter, characterised by waning enthusiasm, drying up of funding and jobs.
Mitchell argued over-optimism among people, the media and even experts arise from fallacies in our understanding of AI and the intuitions about the nature of intelligence. She outlined four major fallacies:
Narrow intelligence and general intelligence
One of the most common fallacies is that narrow intelligence is on a continuum with general intelligence. Narrow intelligence refers to a machine’s ability to perform a single task extremely well. Advances made in narrow AI are often described as the first step towards general AI.
For example, Deep Blue, the chess-playing computer was popularly hailed as the first step in the AI revolution, IBM’s Watson system was described as the entry to a ‘new era of computing’, and most recently, OpenAI’s GPT-3 was called a step towards general intelligence. This is commonly called the ‘first step fallacy’, a term coined by philosopher and mathematician Yehoshua Bar-Hillel. In philosopher Hubert Dreyfus’ words, this term means any improvement in our programs, no matter how trivial, is considered as ‘progress’. Like Dreyfus, Mitchell believes the ‘unexpected obstacle’ in the so-assumed continuum of AI progress has been a problem of common sense.
Easy tasks and hard tasks
Moravec’s paradox, named after roboticist Hans Moravec, states that it is comparatively easy to make computers demonstrate adult level performance on intelligence tests or playing games like chess, but it is impossible for them even to exhibit skills of a toddler when it comes to perception and mobility.
It means that tasks that humans perform almost effortlessly, like making sense of what we see, conversing with another person, or even simply walking without bumping into obstacles, can be some of the hardest tasks to accomplish for machines. Conversely, solving puzzles and complex mathematical problems, translating text between thousands of languages are relatively easier for machines than humans.
Wishful mnemonics
AI is full of ‘wishful mnemonics’ said Mitchell in her paper. She referred to the terms generally associated with human intelligence being used for the evaluation of AI programs. For example, machine learning and deep learning methods are very different from learning in humans or even animals. Similarly, one of the subfields of machine learning is transfer learning which refers to transferring the knowledge they have gained to newer situations. While this capability is fundamental to humans, it is still an open problem for machines.
Source : https://analyticsindiamag.com/ai-winter-is-coming-four-fallacies-in-ai-research/