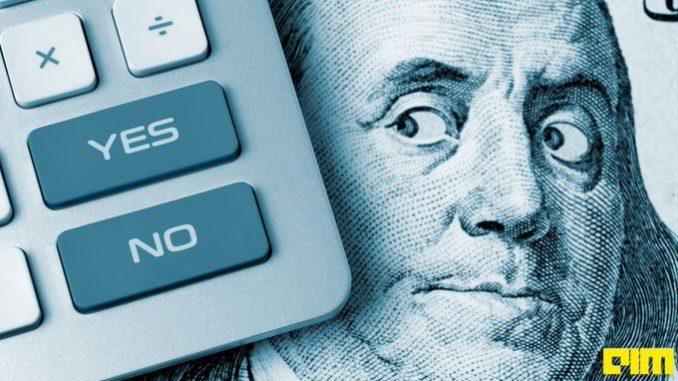
At the ‘Deep Learning DevCon 2021,’ hosted by Analytics India Magazine, a two-day influential conference on deep learning, data science academic lead at Times Professional Learning Raghavendra Nagaraja Rao, touched upon various data imbalances and techniques to enhance the performance of a classification model.
Rao has over 15 years of experience. Before joining Times Professional Learning, a division of Bennett, Coleman & Co. Ltd, he had worked with Manipal Global Education Services as a technical evangelist with expertise in UNIX, SQL, Big Data, data exploration, stats and machine learning, text analytics and NLP.
At the ‘Deep Learning DevCon 2021,’ hosted by Analytics India Magazine, a two-day influential conference on deep learning, data science academic lead at Times Professional Learning Raghavendra Nagaraja Rao, touched upon various data imbalances and techniques to enhance the performance of a classification model.
Rao has over 15 years of experience. Before joining Times Professional Learning, a division of Bennett, Coleman & Co. Ltd, he had worked with Manipal Global Education Services as a technical evangelist with expertise in UNIX, SQL, Big Data, data exploration, stats and machine learning, text analytics and NLP.
What is a Classification Model?
A classification model is a technique that tries to draw conclusions or predict outcomes based on input values given for training. The input, for example, can be a historical bank or any financial sector data. The model will predict the class labels/categories for the new data and say if the customer will be valuable or not, based on demographic data such as gender, income, age, etc.
What is Target Class imbalance?
Target class imbalance is the classes or the categories in the target class that are not balanced. Rao, giving an example of a marketing campaign, said, let’s say we have a classification task on hand to predict if a customer will respond positively to a campaign or not. Here, the target column — responded has two classes — yes or no. So, those are the two categories.