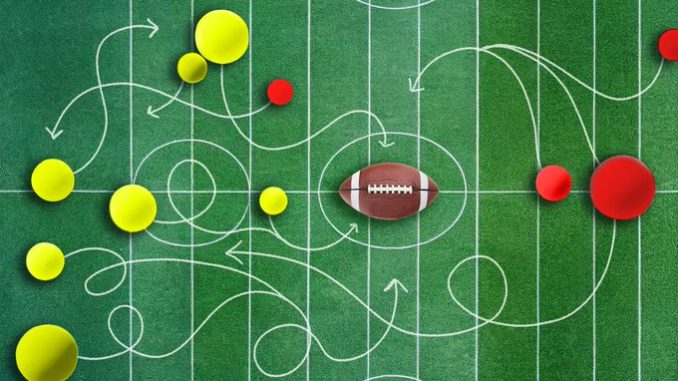
For data science teams to succeed, business leaders need to understand the importance of MLops, modelops, and the machine learning life cycle. Try these analogies and examples to cut through the jargon.
If you’re a data scientist or you work with machine learning (ML) models, you have tools to label data, technology environments to train models, and a fundamental understanding of MLops and modelops. If you have ML models running in production, you probably use ML monitoring to identify data drift and other model risks.
Data science teams use these essential ML practices and platforms to collaborate on model development, to configure infrastructure, to deploy ML models to different environments, and to maintain models at scale. Others who are seeking to increase the number of models in production, improve the quality of predictions, and reduce the costs in ML model maintenance will likely need these ML life cycle management tools, too.
Unfortunately, explaining these practices and tools to business stakeholders and budget decision-makers isn’t easy. It’s all technical jargon to leaders who want to understand the return on investment and business impact of machine learning and artificial intelligence investments and would prefer staying out of the technical and operational weeds.