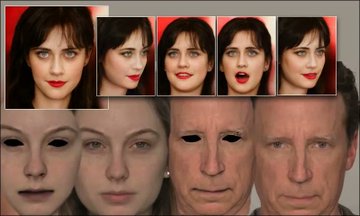
Opinion When Generative Adversarial Networks (GANs) first demonstrated their capability to reproduce stunningly realistic 3D faces, the advent triggered a gold rush for the unmined potential of GANs to create temporally consistent video featuring human faces.
Somewhere in the GAN’s latent space, it seemed that there must be hidden order and rationality – a schema of nascent semantic logic, buried in the latent codes, that would allow a GAN to generate consistent multiple views and multiple interpretations (such as expression changes) of the same face – and subsequently offer a temporally-convincing deepfake video method that would blow autoencoders out of the water.
High-resolution output would be trivial, compared to the slum-like low-res environments in which GPU constraints force DeepFaceLab and FaceSwap to operate, while the ‘swap zone’ of a face (in autoencoder workflows) would become the ‘creation zone’ of a GAN, informed by a handful of input images, or even just a single image.
There would be no more mismatch between the ‘swap’ and ‘host’ faces, because the entirety of the image would be generated from scratch, including hair, jawlines, and the outermost extremities of the facial lineaments, which frequently prove a challenge for ‘traditional’ autoencoder deepfakes.