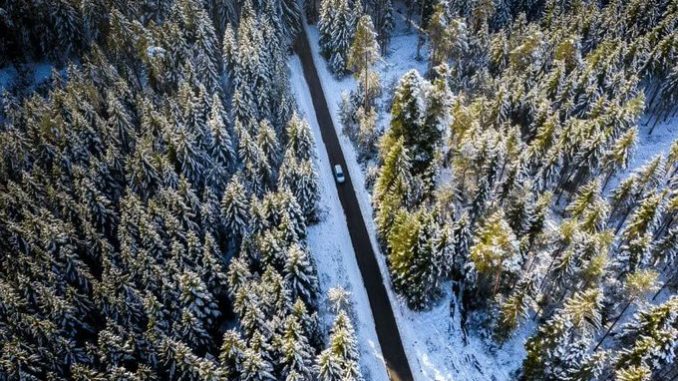
A team of researchers at Cornell University has developed a new method enabling autonomous vehicles to create “memories” of previous experiences, which can then be used in future navigation. This will be especially useful when these self-driving cars can’t rely on sensors in bad weather environments.
Learning From the Past
Current self-driving cars that use artificial neural networks have no memory of the past, meaning they are constantly “seeing” things for the first time. And this is true regardless of how many times they’ve driven the exact same road.
Killian Weinberger is senior author of the research and a professor of computer science.
“The fundamental question is, can we learn from repeated traversals?” said Weinberger. “For example, a car may mistake a weirdly shaped tree for a pedestrian the first time its laser scanner perceives it from a distance, but once it is close enough, the object category will become clear. So, the second time you drive past the very same tree, even in fog or snow, you would hope that the car has now learned to recognize it correctly.”
Led by doctoral student Carlos Diaz-Ruiz, the group created a dataset by driving a car equipped with LiDAR sensors. It was driven around a 15-kilometer loop a total of 40 times over an 18-month period. The various test drives captured different environments, weather conditions, and times of day. All of this created a dataset with more than 600,000 scenes.