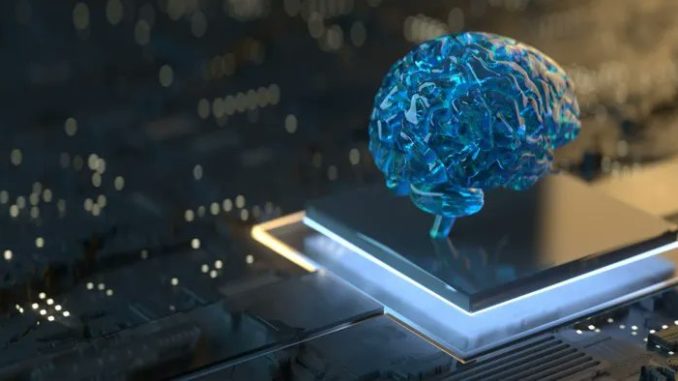
Experts at the National Institute of Standards and Technology want public and private entities to take a socio-technical approach to implementing artificial intelligence technologies to help mitigate algorithmic biases and other risks to AI systems, as detailed in a new playbook.
These recommendations to help organizations navigate the pervasive biases that often accompany AI technologies are slated to come out by the end of the week, Nextgov has learned. The playbook is meant to act as a companion guide to NIST’s Risk Management Framework, the final version of which will be submitted to Congress in early 2023.
Reva Schwartz, a research scientist and principal AI investigator at NIST, said that the guidelines act as a comprehensive, bespoke guide for public and private organizations to tailor to their internal structure, rather than function as a rigid checklist.
“It’s meant to help people navigate the framework, and implements practices internally that could be used,” Schwartz told Nextgov. “The purpose of both the framework and the playbook is to get better at approaching the problem and transforming what you do.”
She said that, along with proactively identifying other risks, the playbook was created to underscore specific ways to prevent bias in AI technology, but veers away from a rigid format so as to work for a diverse number of firms.
“We won’t ever tell anybody, ‘this is absolutely how it should be done.’ We’re gonna say, ‘here’s the laundry list of things…here’s some best practices,’” Schwartz added.
A key feature the playbook looks to impart is ensuring there is a strong element of human management behind AI systems. This is the fundamental principle of the socio-technical approach to managing technology: being aware of the human impact on technology to prevent it from being used in ways designers did not initially intend.
Schwartz noted that NIST has been working on controlling for three types of biases that emerge with AI systems: statistical, systemic and human.