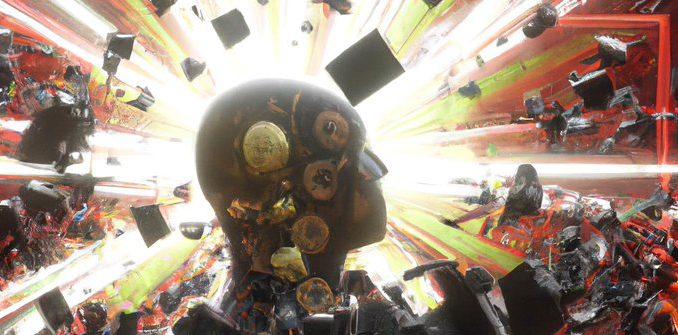
How would an artificial intelligence (AI) decide what to do? One common approach in AI research is called “reinforcement learning”.
Reinforcement learning gives the software a “reward” defined in some way, and lets the software figure out how to maximise the reward. This approach has produced some excellent results, such as building software agents that defeat humans at games like chess and Go, or creating new designs for nuclear fusion reactors.
However, we might want to hold off on making reinforcement learning agents too flexible and effective.
As we argue in a new paper in AI Magazine, deploying a sufficiently advanced reinforcement learning agent would likely be incompatible with the continued survival of humanity.
The reinforcement learning problem
What we now call the reinforcement learning problem was first considered in 1933 by the pathologist William Thompson. He wondered: if I have two untested treatments and a population of patients, how should I assign treatments in succession to cure the most patients?
More generally, the reinforcement learning problem is about how to plan your actions to best accrue rewards over the long term. The hitch is that, to begin with, you’re not sure how your actions affect rewards, but over time you can observe the dependence. For Thompson, an action was the selection of a treatment, and a reward corresponded to a patient being cured.
The problem turned out to be hard. Statistician Peter Whittle remarked that, during the second world war,