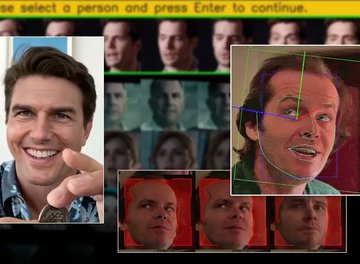
Excluding ‘traditional’ CGI methods, which date back to the 1970s, there are currently three mainstream AI-based approaches to creating synthetic human faces, only one of which has attained any widespread success or societal impact: autoencoder frameworks (the architecture behind current viral deepfakes); Generative Adversarial Networks (GANs); and Neural Radiance Fields (NeRF).
Of these, NeRF — a late entrant that’s also capable of recreating the entire human form — is at the most rudimentary stage in terms of its facial generation capabilities; GANs can create the most convincing faces, but are still too volatile and ungovernable to easily output realistic video footage; and autoencoder frameworks, which have captivated (and, arguably, menaced) the world, require ‘host’ footage, and are largely confined to the inner areas of the face, which adds the further burden of finding a ‘target’ who closely resembles the ‘injected’ identity.