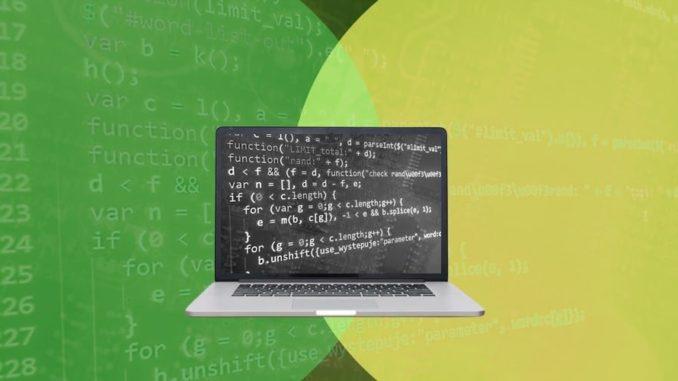
To say that it’s challenging to achieve AI at scale across the enterprise would be an understatement.
An estimated 54% to 90% of machine learning (ML) models don’t make it into production from initial pilots for reasons ranging from data and algorithm issues, to defining the business case, to getting executive buy-in, to change-management challenges.
In fact, promoting an ML model into production is a significant accomplishment for even the most advanced enterprise that’s staffed with ML and artificial intelligence (AI) specialists and data scientists.
Enterprise DevOps and IT teams have tried modifying legacy IT workflows and tools to increase the odds that a model will be promoted into production, but have met limited success. One of the primary challenges is that ML developers need new process workflows and tools that better fit their iterative approach to coding models, testing and relaunching them.
That’s where MLOps comes in: The strategy emerged as a set of best practices less than a decade ago to address one of the primary roadblocks preventing the enterprise from putting AI into action — the transition from development and training to production environments.
Gartner defines MLOps as a comprehensive process that “aims to streamline the end-to-end development, testing, validation, deployment, operationalization and instantiation of ML models. It supports the release, activation, monitoring, experiment and performance tracking, management, reuse, update, maintenance, version control, risk and compliance management, and governance of ML models.”