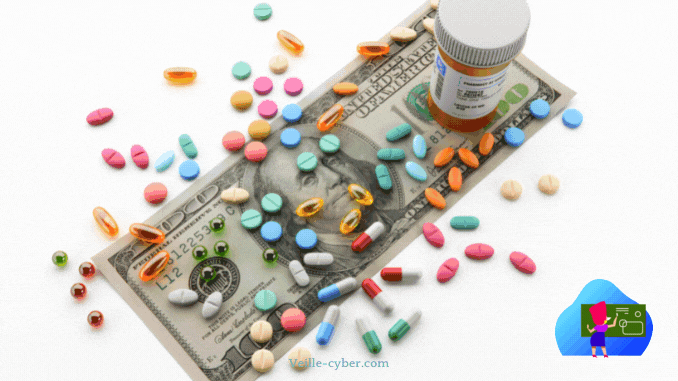
The healthcare industry is becoming more and more interested in machine learning. In this article, we’ll look at some of the healthcare applications of ML-based solutions and their benefits.
The problems that occur in healthcare are often complex and challenging to describe. On the one hand, the dynamic development of medicine encourages the search for new models of diagnostic and therapeutic procedures. Still, on the other hand, ever-larger datasets make it difficult to explore and analyze.
Hence, the healthcare industry is becoming more and more interested in machine learning. In this article, we’ll look at some of the healthcare applications of ML-based solutions and their benefits.
Machine learning is a rapidly growing field of research with a great future. This part of AI science plays one of the most significant roles in many areas of our lives, including healthcare. For example, the number of patients in hospitals is proliferating, which means that it is becoming increasingly difficult to analyze and record all patient data.
Machine learning offers an excellent solution to this problem: It facilitates the automation of data analysis and strengthens the healthcare system. Machine learning applications in healthcare also include disease detection and diagnosis, drug discovery, and personalized medicine.
The purpose of implementing ML solutions in healthcare
The ultimate goal of machine learning is to make machines and algorithms more efficient and reliable. In the healthcare industry, the machine’s job is not to replace the doctor but rather to help them provide better service and care. Therefore, let’s take a look at individual examples of the use of machine learning in healthcare.
- Personalization of treatment
One of the most important areas of machine learning in healthcare is personalized treatment. The goal of personalized therapy is to improve individual health services using very personal data and analysis techniques. Machine learning tools for calculations and statistics develop customized treatment systems based on patients’ genetic information and symptoms.
In addition, supervised machine learning algorithms allow the creation of personalized treatment systems using the medical information of individual patients.
Medicine has come a long way, from a generalized approach to treatment with broad-spectrum antibiotics to treating disease and a preventive process that considers the individual variability of each person’s genes, environment, and lifestyle.
By combining clinical, pharmaceutical, and socioeconomic data with machine learning algorithms, researchers can observe efficacy patterns in individual treatments and identify genetic variations that may be correlated with disease, success, or failure.
- Health service management
From routine front office automation and reporting to the analysis of pharmaceutical marketing research, machine learning thrives in many areas of operations and management. However, today, the journey within the hospital generates many administrative tasks with different processes impacting doctors, nurses, and patients, which complicate the trip and cause high costs.
Machine learning is being used by doctors and healthcare staff increasingly using artificial intelligence to automate these administrative tasks to simplify patient travel to hospital, improve care, re-focus medical staff on their medical studies, or optimize costs.
- Virtual assistants supporting patients
Virtual assistants are an increasingly popular solution in the healthcare sector via chatbots or dedicated applications. In particular, we see the emergence of machine learning-based virtual assistants to support patients in all pathways of care.
There is a trend towards virtual nurses who are always available, answering patients’ questions immediately, and providing advice. Such virtual assistants can record interactions and disease records so that physicians can use them in their treatment.
In addition to supporting the patient, the goal of these virtual assistants is to generate substantial savings by limiting, i.a., hospital visits or stress related to medical care.
- Development of new drugs
The use of machine learning in preliminary (early stage) drug discovery has the potential for various services, from the initial screening of drug compounds to the expected success rate based on biological factors. In addition, it can potentially help predict the efficacy and safety of drugs (which is one of the main goals of new drug development) and provide information about fresh ingredients that may become new drugs.
This new approach to drug development is necessary because current guidelines assume that each compound has a specific and well-researched purpose, limiting drug development to small groups of compounds. Further, the assumption has been that the “best” combination is the first to reach clinical trials.
- Diagnoses
In the care process, the diagnostic phase is essential for patient follow-up. Machine learning provides healthcare professionals with new solutions to save time and optimize correct diagnoses. In addition, it opens up new perspectives in the diagnosis and prediction of many diseases, including:
- Heart disease diagnosis: scientists are developing machine learning algorithms to help diagnose heart disease. It is a topic that has been the subject of much research worldwide, and an automated heart disease diagnostic system would be one of the most outstanding human achievements of the 21st century.
- Predicting diabetes: diabetes can damage various parts of the body, such as the heart, kidneys, and nervous system. Machine learning is supposed to detect markers of diabetes early enough to save patients’ lives. Medical staff can use many algorithms to predict diabetes.
- Predicting liver disease: Concepts of data mining and machine learning have recently emerged to search for a system to predict liver disease. Trying to predict liver disease is a real challenge, partly because there are so many possible diseases that can affect the liver and also because there is a considerable amount of data on liver disease. However, scientists are doing everything in their power to get around these problems.
- Cancer Detection: Machine learning can see and detect different types of cancer. Since the inception of AI, scientists have greatly improved the algorithms that can now describe the tumors observed and detect more diverse forms of cancer. These improvements now allow AI to detect tumors with incredible precision, all without being influenced by elements that may confuse medical experts, for example, air bubbles or hemorrhages.
Conclusion
Machine learning applications in healthcare help physicians and researchers develop and deliver personalized therapy, improve quality of life, and detect many diseases early. Machine learning promises advancements in healthcare so extensive that perhaps we even cannot imagine them fully today.
For more information, check https://addepto.com/machine-learning-consulting/