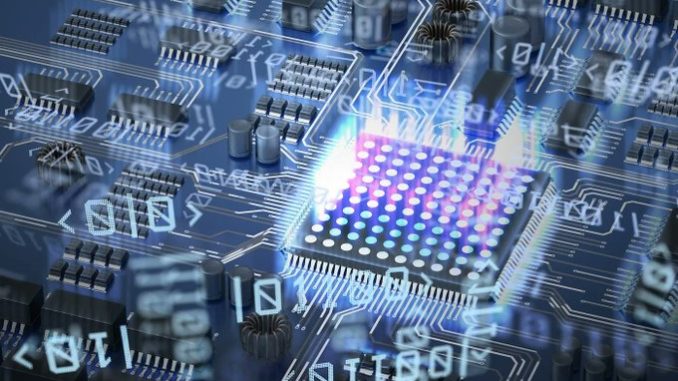
Quantum physics promises big changes for many fields, including machine learning—in theory, at least. In reality, quantum machine learning is still largely theoretical and has a long way to go before it becomes practical for widespread application.
When and if quantum computers become more feasible and accessible, they will greatly increase the speed of machine learning processing and open a lot of possibilities for new types of machine learning. Therefore, it’s important to understand what quantum machine learning is, how it theoretically works, how it compares to classical machine learning, and what challenges lie in store for the future of quantum ML.
What is quantum machine learning?
To grasp what quantum machine learning is, we first need to understand machine learning and quantum mechanics separately.
Machine learning (ML) is a subset of artificial intelligence (AI) and computer science that uses data, algorithms, and software to imitate the way that humans learn knowledge over time. On the other hand, quantum computing is a cutting-edge technology field that uses the principles of quantum mechanics to solve problems that are too complicated for classical computers to handle.
Thus, quantum machine learning effectively combines these two fields to create computers that can operate and learn at a scale far beyond that of “ordinary” machine learning