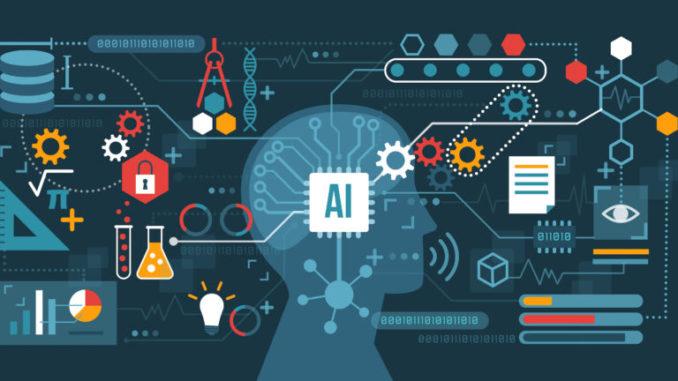
For years, we’ve been aware that AI is set to be one of the world’s biggest – if not the biggest – technological and economic game-changers. With PwC estimating that by 2030 AI will grow the global economy by nearly $16 trillion, we’ve become used to claims that it will be a transformative technology from the media. For those of us who actually work with AI though, it’s clear that some of this optimism needs to be tempered. That’s because right now many of the processes to develop, test, deploy, and monitor AI models are not as efficient as they could be.
In practice, most people who’ve worked with AI or ML in industry know that the technology requires a great deal of manual intervention to be able to smoothly run in a production environment. To take one example, the data scientists who help develop and train models end up finding most of their time consumed on manual and repetitive tasks around data preparation – around 45% of their working hours. By contrast, the real value-add part of their job – model training, scoring, and deployment – only consumes 12% of a data scientist’s working time.
10 Predictions For Blockchain, Crypto Assets, DeFi, And NFTs For 2022