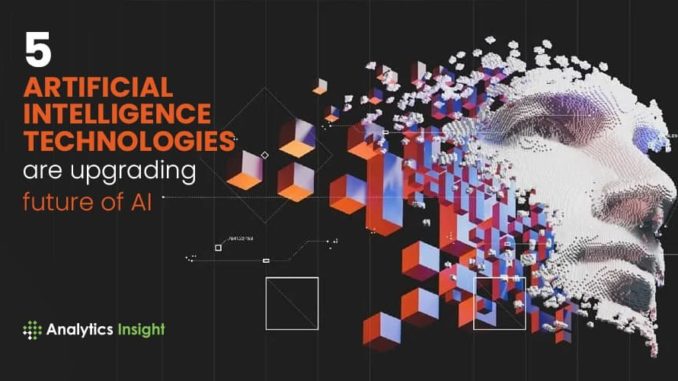
The replication of human intellectual processes by machines, particularly computer systems, is known as artificial intelligence. Expert systems, natural language processing, speech recognition, and machine vision are examples of AI applications. Artificial Intelligence is extensively utilized to give consumers customized suggestions based on their prior searches and purchases, as well as other online activities. In business, AI plays a critical role in product optimization, inventory planning, and logistics, among other things. In 1956, the phrase “artificial intelligence” was coined.
Artificial Intelligence and Its Applications:
- Health-care Industry: Artificial intelligence in health care can give tailored medication and X-ray readings. Personal health care assistants can serve as life coaches, reminding you to take your medications, exercise, and eat more healthily.
- Manufacturing: Manufacturing AI may utilize recurrent networks, a form of deep learning network used with sequence data, to evaluate factory IoT data as it flows in from connected equipment to anticipate projected load and demand.
- Life Sciences: AI technology can unlock the full potential of data to tackle some of our largest health issues, from guaranteeing medication safety to bringing novel medicines to market faster.
- Retail: Virtual shopping features provided by Retail AI include tailored suggestions and discussion of purchasing choices with the customer. Artificial intelligence will also boost stock management and site layout technology.
- Banking sector: Artificial intelligence in banking improves the speed, precision, and efficacy of human activities. AI approaches may be utilized in financial institutions to determine which transactions are likely to be fraudulent, implement quick and accurate credit scoring, and automate labor-intensive data management activities.
- Public Sector: Artificial intelligence has the potential to make smart cities smarter. It can help the military with mission readiness and preventative maintenance. AI has the potential to increase program efficiency and effectiveness across the board.
Machine Learning:
Machine learning is a type of data analysis that automates the creation of analytical models. It’s a field of Artificial intelligence based on the notion that computers can learn from data, recognize patterns, and make choices with little or no human input.
Applications of Machine Learning: The importance of machine learning technology has been acknowledged by most sectors that deal with huge volumes of data.
- Services in the financial sector: Machine learning is used by banks and other financial institutions for two main purposes: identifying valuable insights in data and preventing fraud.
- Health-care services: Because of the development of wearable gadgets and sensors that can utilize data to analyze a patient’s health in real-time, machine learning is a fast-growing trend in the healthcare sector. Medical specialists may use the technology to examine data and spot trends or red flags that could lead to better diagnosis and treatment.
- Government: Because they have many sources of data that may be mined for insights, government organizations such as public safety and utilities have a special need for machine learning.
- Retail: Machine Learning is used to evaluate your purchasing history by retail websites that propose goods you might enjoy based on prior purchases. Machine learning is used by retailers to collect, evaluate, and apply data to customize shopping experiences, execute marketing campaigns, pricing optimization, item supply planning, and consumer insights.
Deep Learning:
Deep learning is a sort of machine learning in which a computer is taught to do human-like tasks such as speech recognition, picture identification, and prediction. Deep learning sets up fundamental parameters about the data and educates the computer to learn on its own by detecting patterns utilizing multiple layers of processing, rather than arranging data to run through predetermined equations.
Applications of Deep Learning:
- Speech recognition: Deep learning for voice recognition has gained traction in both the corporate and academic realms. To detect human speech and voice patterns, Xbox, Skype, Google Now, and Apple’s Siri, to name a few, are already using deep learning technology in their systems.
- Natural language processing: For many years, neural networks, a key component of deep learning, have been utilized to process and interpret the textual text. This approach, which is a subset of text mining, may be used to find patterns in a variety of sources, including consumer complaints, medical notes, and news stories, to name a few.
- Recognition of images: Automatic picture captioning and scene description are two practical applications of image recognition. The adoption of 360-degree camera technology in self-driving automobiles will also enhance picture recognition.
- Systems of recommendation: Amazon and Netflix popularized the idea of a recommendation system that can predict what you might be interested in next based on your previous activity. Deep learning may be used to improve suggestions across many platforms in complicated contexts like music tastes or apparel preferences.
Natural Language Processing (NLP):
Natural language processing (NLP) is an Artificial intelligence field that aids computers in comprehending, interpreting, and manipulating human language. To bridge the gap between human communication and machine comprehension, NLP depends on a variety of fields, including computer science and computational linguistics. Natural language processing isn’t a new subject, but it’s progressing quickly thanks to a growing interest in human-machine communication, as well as the availability of huge data, powerful computation, and improved algorithms.
Applications of Natural Language Processing:
- Text analytics and natural language processing: Text analytics, which counts, classifies, and categorizes words to extract structure and meaning from enormous quantities of material, goes hand in hand with Natural language processing.
- Examples of NLP in everyday life: NLP has a wide range of common and practical applications in our daily lives. Bayesian spam filtering is a statistical natural language processing approach that compares spam terms to legitimate emails to identify spam. Have you ever missed a phone call and then read the voicemail transcript in your email inbox or on your smartphone app? That’s speech-to-text conversion, which is an NLP feature.
Computer Vision:
Computer vision is an artificial intelligence area that teaches computers to analyze and comprehend images. Machines can reliably detect and categorize things using digital pictures from cameras and videos, as well as deep learning models, and then react to what they observe. In many areas, computer vision approaches and surpasses human visual ability, from identifying people to analyzing the live action of a football game.
Applications of Computer Vision:
- Picture segmentation divides an image into numerous areas or fragments, each of which may be studied independently.
- Object detection is the process of identifying a specific object in a photograph. A football field, an offensive player, a defensive player, a ball, and so on are all recognized using advanced object identification in a single image. To build a bounding box and identify everything inside it, these models employ an X, Y coordinate.
- Facial recognition is a form of sophisticated object detection that not only recognizes but also identifies a specific person in a picture.
- Edge detection is a method for determining the outside edge of an item or landscape to better identify what is in the image.
- The technique of identifying repeating forms, colors, and other visual cues in pictures is known as pattern detection.
- The categorization of photographs divides them into distinct categories.
- Feature matching is a form of pattern recognition that compares picture similarities to help categorize them.