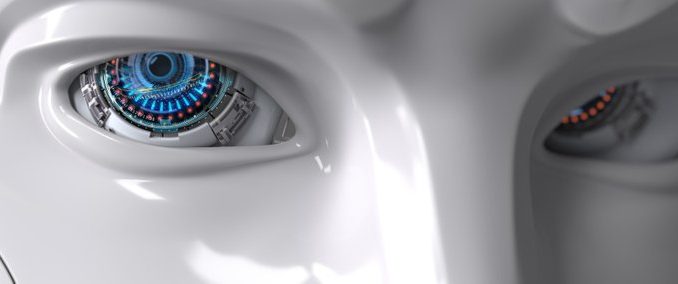
Good vs. evil – a tale as old as time – but there is always a gray area, especially when it comes to modern AI (Artificial Intelligence) technology
The recent objections to using A.I.-generated audio to mimic Anthony Bourdain’s voice without disclosing it to viewers in a new documentary is one such example of this tale. Most revolutionary technology can be used for good or bad, and the deepfake like the one used for Bourdain is no exception. Yet, we continue to give the malicious actors the spotlight rather than focusing on the benefits of AI. Why is this?
A Deepfake, or synthetic media in which a person in an existing image or video is replaced by a computer-generated version, is powered by a host of complex and technical emerging technologies including generative networks, neural rendering and cinematic VFX. All of these technologies have the power to transform how AI systems are built. One of the first truly viral deepfake examples was with none other than Tom Cruise, which launched a lot of conversation around ethics of AI technologies, deepfakes, and what the future of facial recognition and computer vision means for society.
On the flip side — and the side not looked at nearly enough — these technologies can be used for positive, innovative practices that can actually cut-down on safety and privacy concerns. .
One of these technologies is called synthetic data, which has the ability to train these AI systems in a safe, ethical way for a fraction of the cost. For reference, traditional AI systems are built using human annotated real-data. Synthetic data instead aims to simulate real-world scenarios to help train computer vision systems virtually. For instance, to train AI systems for autonomous vehicles one can leverage visual effects technologies to simulate city streets, pedestrians, traffic patterns, and the weather. Synthetic data of people in different environments can be used to help build better mobile phone face unlock systems, smart home products, teleconferencing apps, security applications, and much more. Synthetic data provides a way to have unlimited, perfectly labeled data at minimal costs compared to manually labeled data. Synthetic data can help reduce the bias often seen in traditional AI data sets due to non-representative real-world data, enhance privacy and play a pivotal role in the democratization of access to AI.
Diving into three positive use cases for synthetic data via computer vision will help explain its beneficial use in the real world which deserve more spotlight:
● Bias Reduction in Identity Verification. We routinely unlock our phones using identity verification AI systems. Financial, legal, government, healthcare and other institutions are increasingly using facial recognition for identity verification to improve user-experience and decrease fraud. Yet existing data sets used to train these systems are commonly built using data that does not properly represent age, gender, and skin color – not to mention contain inconsistent lighting variations and camera angles. Without proper training data, the models are biased and tend to perform poorly for underrepresented demographics. This raises key ethical issues that need to be addressed. Synthetic data reduces bias by allowing AI scientists to create balanced datasets equally representing groups. It instantly helps organizations meet regulatory and compliance requirements, and helps organizations build more fair and ethical AI systems.
● Privacy Preservation for Smart Home Products. The next wave of smart home and smart assistant products will include cameras that understand the actions of people inside their homes. This presents an opportunity for smart technology to truly distinguish between a variety of objects, recognize human behavior, and see how humans interact with said objects. Building these systems is complicated by privacy concerns around using data of people especially within their homes. With synthetic data, highly diverse indoor virtual environments with simulated human models can be created. Training with synthetic data alleviates privacy issues.
● Data Democratization for Driver Safety. Car manufacturers require massive data sets to build truly autonomous vehicles that are safe and secure. From understanding unexpected weather conditions to a small child jumping in the street, a car’s intelligent sensing configurations that make autonomous decisions need to be programmed to anticipate a broad set of environments and solutions without compromising privacy. Synthetic data enables all manufacturers to scale up data quickly, which democratizes innovation in the field and ultimately leads to safer vehicles for all of us.
The news cycle around Anthony Bourdain’s deepfake is just one example of the ethical issues around emerging AI technology. However, the underlying technologies have the potential for significant positive impact. Using synthetic data to train more AI systems, can reduce bias, safeguard consumer privacy and democratize access to data needed to build new AI products. As synthetic data is further adopted, the world is bound to see further benefits in a number of areas and applications.
Source : https://technative.io/good-vs-evil-with-modern-ai-whats-the-solution/