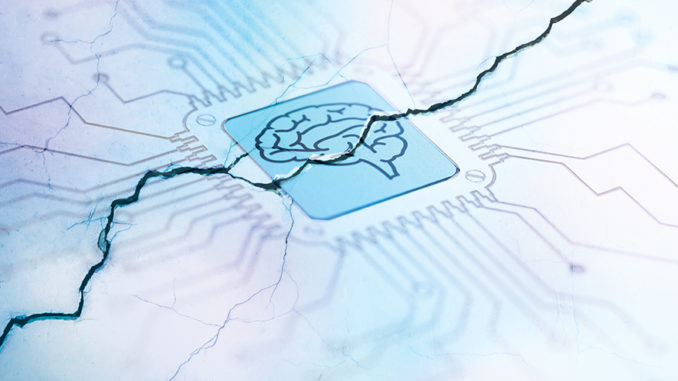
As researchers and engineers race to develop new artificial intelligence systems for the U.S. military, they must consider how the technology could lead to accidents with catastrophic consequences.
In a startling, but fictitious, scenario, analysts at the Center for Security and Emerging Technology — which is part of Georgetown University’s Walsh School of Foreign Service — lay out a potential doomsday storyline with phantom missile launches.
In the scenario, U.S. Strategic Command relies on a new missile defense system’s algorithms to detect attacks from adversaries. The system can quickly and autonomously trigger an interceptor to shoot down enemy missiles which might be armed with nuclear warheads.
“One day, unusual atmospheric conditions over the Bering Strait create an unusual glare on the horizon,” the report imagined. The missile defense system’s “visual processing algorithms interpret the glare as a series of missile launches, and the system fires interceptors in response. As the interceptors reach the stratosphere, China’s early-warning radar picks them up. Believing they are under attack, Chinese commanders order a retaliatory strike.”
Doomsday examples such as this illustrate the importance of getting artificial intelligence right, according to the report, “AI Accidents: An Emerging Threat — What Could Happen and What to Do.”
While AI accidents in other sectors outside of the Defense Department could certainly be catastrophic — say, with power grids — the military is a particularly high-risk area, said Helen Toner, co-author of the report and CSET’s director of strategy.
“The chance of failure is higher and obviously when you have weaponry involved, that’s always going to up the stakes,” she said during an interview.
AI failures usually fit into three different categories, according to the report. These include robustness, specification and assurance.
Failures of robustness occur when a system receives abnormal or unexpected inputs that cause a malfunction, the report said. Failures of specification happen when a system attempts “to achieve something subtly different from what the designer or operator intended, leading to unexpected behaviors or side effects.” Failures of assurance occur when a system cannot be adequately monitored or controlled during operation.
While the military could face any of those types of accidents, Toner noted that robustness is a top concern.
“All of them come into play,” she said. “Robustness is an especially big challenge because … [of] the presence of an adversary who’s going to try to cause your systems to fail.”
However, the military is institutionally attuned to such dynamics, so officials would likely put the most attention and resources toward addressing those types of issues, she added.
The Pentagon’s Joint Artificial Intelligence Center is actively working to avoid AI accidents and blunders.
Jane Pinelis, the JAIC’s chief of test and evaluation, said the organization has partnered extensively with the Office of the Director of Operational Test and Evaluation as it develops new technology.
“We work with them on a variety of issues,” she said during a recent briefing with reporters. “We work with them and coordinate with them probably a few times every week.”
Each of the JAIC’s systems is tested, and the center is currently collaborating with DOT&E on different systems that are in various stages of development, she said.
However, Toner noted that rigorous testing and evaluation of any deep learning system remains a big challenge. “This isn’t a DoD problem, this is a whole world problem.”
The Defense Department must also be mindful that while researchers and engineers are developing new systems that could one day be more resilient, many of today’s systems will be around for a long time, the CSET report noted.
“Governments, businesses and militaries are preparing to use today’s flawed, fragile AI technologies in critical systems around the world,” the study said. “Future versions of AI technology may be less accident-prone, but there is no guarantee.”
It continued: “The machine learning models of 2020 could easily still be in use decades in the future, just as airlines, stock exchanges and federal agencies still rely today on COBOL, a programming language first deployed in 1960.”
For the Pentagon — which has decades-old equipment in its inventory — that could be particularly problematic, Toner said.
“The Defense Department [has] to be really forward leaning in thinking about ways to use these technologies and then testing them and starting to consider potential use cases,” she said. Officials need “to be really cautious and deliberate in actually embedding them into platforms because … any platform that is acquired is likely to be in use for decades.”
While some issues may become evident early in the development process, others could take years to manifest after systems are already in widespread use, she added.
To better avoid accidents, the federal government should facilitate information sharing about incidents and near misses; invest in AI safety research and development; fund artificial intelligence standards development and testing capacity; and work across borders to reduce accident risks, the report said.
For the military, working across borders is critical, Toner said.
In a defense setting, “a really key component of that is thinking about the strategic stability implications of increasing the use of AI,” she said. Toner seconded recommendations from the National Security Commission on AI — which released its final report to Congress in March — that called for more discussion around crisis dynamics, conflict escalation and strategic stability with Russia and China.
Topics: Robotics, Robotics and Autonomous Systems