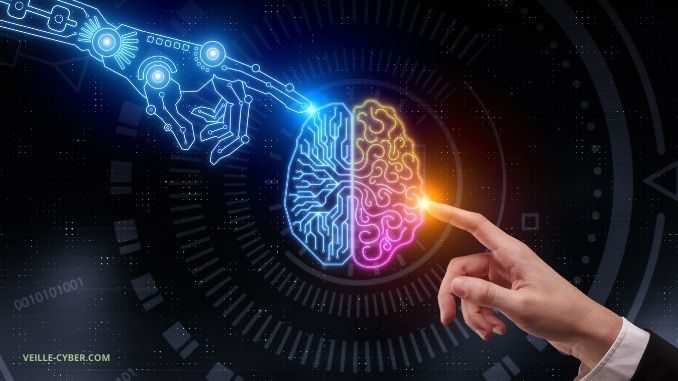
Life sciences companies need the right digital infrastructure, data management practices and cyber readiness to successfully scale up their use of artificial intelligence (AI) tools.
Addressing digital infrastructure needs and issues of data and cyber at the outset will be vital to life sciences and healthcare companies as they increasingly look to AI to streamline clinical and non-clinical processes, improve the diagnosis of diseases, and speed up the development of new medicines and other treatments and develop policies to guide the ethical use of the technology in practice. These are themes we explored in partnership with Intel and the Digital Leadership Forum at a recent event.
Digital infrastructure
When looking at scaling up their use of AI systems, life sciences companies need to consider whether their existing digital infrastructure is fit to support the use of the technology in practice.
Simon Colvin
Partner, Head of Technology, Media and Telecoms
The infrastructure needs to be scalable to meet long-term needs, and resilient to address the heightened risk of collecting data that is of potential value to others
As well as being able to support capability requirements now and in the near future, the infrastructure needs to be scalable to meet long-term needs, and resilient to address the heightened risk of collecting data that is of potential value to others who may get their hands on it.
It is likely that flexible cloud-based systems will be popular options to allow for scalable storage and contingencies as demand grows over time. That said, migrating from on-premises technology to systems operated by third parties, such as cloud providers, is a major undertaking that requires due diligence, strong project management skills and contractual clarity on important issues such as service levels and regulatory compliance – including ensuring appropriate systems and processes to manage personal data and necessary protections against data breaches.
Other challenges will arise if life sciences companies elect to bolt on new AI systems to legacy infrastructure they operate, not least the challenge of delivering scalability and in ensuring the respective systems are interoperable. Often data held in different systems will be in different formats. It will be vital to ensure that there is interoperability of data across systems and platforms.
It will be prudent for life sciences companies exploring how they might implement AI to conduct a review of their existing technology platforms to better understand their capabilities and limitations. This will enable an informed decision to be made about what digital infrastructure is required to support their planned AI scale-up.
Data
AI tools are only as good as the data on which they are trained. The results delivered by AI tools primarily rely on the data that is input. It is therefore essential in scaling the use of AI that sufficient data that is reliable and representative is collected and used. The source of the data will be important to the verification of its integrity and reliability. In the life sciences sector in particular it will be important that the data is representative, both by ensuring sufficient volume of data and the demographic spread, and ensuring the data is not biased.
Cerys Wyn Davies
Partner
To gain patient trust it is essential that there is sufficient transparency as to the purpose and objectives of the use to which the personal data will be put
It is important for the collection of representative health data that patients have trust in the organisations which collect their data and understand the purposes for which their data is collected to unlock the availability of representative data. To gain patient trust it is essential that there is sufficient transparency as to the purpose and objectives of the use to which the personal data will be put, as well as assurances concerning its confidentiality, security and the period of its retention.
Bias can also occur from the selection of the AI training data that is input by AI developers and/or in their further development of the AI algorithms. A diverse AI developer team, informed as to the risks of insufficient or unrepresentative data and of bias, should be deployed. Additionally, AI itself may “learn” to confirm that it is processing sufficient and representative data.
As well as data collection, good data management will also be essential, whether handling personal data, anonymised or aggregated data. Monitoring and auditing data usage from collection and use of training data and other input data through to AI model usage and outputs will support accountability and trust.
Addressing cyber risk
Because the life sciences sector is at the forefront of scientific innovation it is already an attractive target for cyber criminals, including state-sponsored attackers. This means the cyber risks all businesses face – business interruption, costs, potential data loss, regulatory action and reputational harm, to name a few – are heightened in life sciences.
Some attacks are intended to delay, disrupt or undermine trust in critical research projects and cause economic and social harm. Other attacks tend to be focused on stealing personal data, valuable research data and other intellectual properly.
Stuart Davey
Partner
Measures such as the pseudonymisation or full anonymisation of data can help address some of the risks that might arise in the event that data is compromised
One of the central risks comes from the sheer complexity of AI systems. An AI model is heavily reliant upon a number of input assets and component parts, each distinct from one another and dependent on different suppliers. Getting a handle on these assets is vital to understanding the threat landscape and therefore taking action to protect against and prepare for attacks.
The ENISA report, among other things, highlights the risks of hackers gaining unauthorised access to the data that is fed into AI systems and corrupting, “poisoning” or “tampering” with it.
Where this data is personal data such a data breach might be notifiable to regulators under data protection law, and we are seeing a growing trend of data protection-related claims being brought in litigation. However, where AI systems are used to inform decisions on the safety and efficacy of new medicines or medical devices, arguably the biggest potential risk from data being manipulated by hackers is to patient health, where their activity goes undetected.
Life sciences companies looking to scale up their use of AI will want to ensure that those new tools are secure-by-design. Measures such as the pseudonymisation or full anonymisation of data can help address some of the risks that might arise in the event that data is compromised.
While AI is being used by hackers to accelerate and automate attacks and preserve the anonymity of attackers, there is also an opportunity for life sciences companies to fight back by using AI to boost their cyber defences.
For example, AI is capable of detecting and tracking more than 10,000 active phishing sites, as well as reacting and remediating incidents considerably more quickly than humans, while insights from AI-based analysis can be used to design and enhance cybersecurity policies and procedures.
AI can offer proactive threat prevention.
Co-written by Simon Colvin of Pinsent Masons.