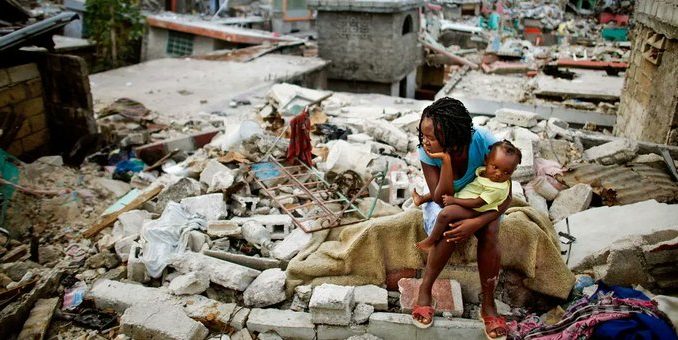
The model could uncover quakes that would previously have been dismissed as human-generated vibrations.
Cities are loud places. Traffic, trains, and machinery generate a lot of noise. While it’s a mere inconvenience much of the time, it can become a deadly problem when it comes to detecting earthquakes. That’s because it’s difficult to spot the telltale signal of an approaching earthquake in seismic sensor data amid the general human-generated vibrations typical of bustling cities, known as urban seismic noise.
Researchers from Stanford have found a way to get a clearer signal. They’ve created an algorithm, described in a paper in Science Advances today, that they claim improves the detection capacity of earthquake monitoring networks in cities and other built-up areas. By filtering out urban seismic noise, it can boost the overall signal quality and recover signals that may have previously been too weak to register.
Algorithms trained to sift out urban seismic noise could be of particular use to monitoring stations in and around bustling earthquake-prone cities in South America, Mexico, the Mediterranean, Indonesia, and Japan.