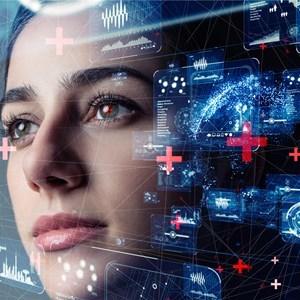
The changing dynamics of the digital world have led to several privacy challenges for businesses, large and small. This is placing increasing pressure on them to evolve their processes and strategies. Much of the burden stems from the sheer volume of data present today, and in fact, the volume of data is predicted to balloon to 175 zettabytes (ZB) by 2025. Today, it is simply beyond human capability to effectively process and protect privacy without the assistance of privacy-enhancing technologies (PETs).
This has led to an explosion of adaptive machine learning (ML) algorithms that can wade through the mountain of data while continuously and efficiently changing their behavior in real-time as new data streams are fed into them. However, while ML is key to leveraging and learning from big data at scale, it can create privacy challenges. In fact, traditional ML requires data to be stored on a centralized server for analysis, including transporting data to cloud environments; this opens the doors to a plethora of security and privacy implications.