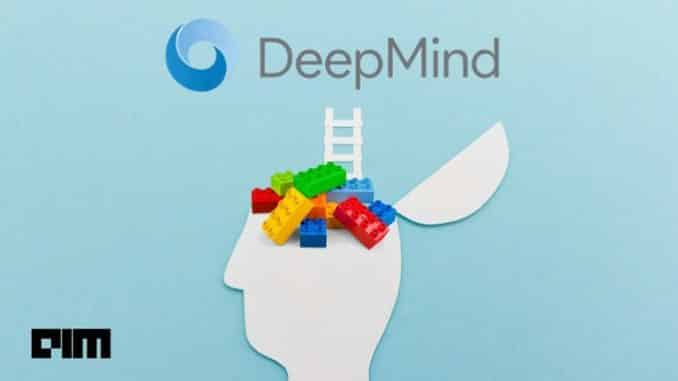
In its latest step towards general-purpose AI systems, DeepMind has proposed XLand, a virtual environment, to formulate new learning algorithms, which control how agent trains and the games on which it trains. XLand was introduced via a paper titled, “Open-Ended Learning Leads to Generally Capable Agents“, in which DeepMind researchers demonstrated a technique to train an agent capable of playing many different games without requiring human interaction data
Challenges with traditional reinforcement learning
The repetitive process of trial and error has proven effective in teaching computer systems to play many games, including chess, shogi, Go, and StarCraft II. However, one of the main challenges with reinforcement learning-trained systems is a lack of training data. Systems trained by reinforcement learning are unable to adapt their learned behaviours to new tasks because they are not trained on a broad enough set of tasks.