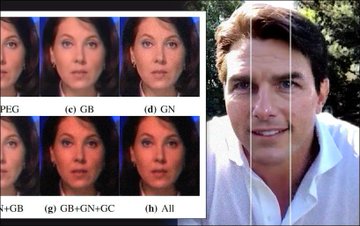
Not all deepfake practitioners share the same objective: the impetus of the image synthesis research sector – backed by influential proponents such as Adobe, NVIDIA and Facebook – is to advance the state of the art so that machine learning techniques can eventually recreate or synthesize human activity at high resolution and under the most challenging conditions (fidelity).
By contrast, the objective of those who wish to use deepfake technologies to spread disinformation is to create plausible simulations of real people by many other methods than the mere veracity of deepfaked faces. In this scenario, adjunct factors such as context and plausibility are almost equal to a video’s potential to simulate faces (realism).
This ‘sleight-of-hand’ approach extends to the degradation of final image quality of a deepfake video, so that the entire video (and not just the deceptive portion represented by a deepfaked face) has a cohesive ‘look’ that’s accurate to the expected quality for the medium.
‘Cohesive’ doesn’t have to mean ‘good’ – it’s enough that the quality is consistent across the original and the inserted, adulterated content, and adheres to expectations. In terms of VOIP streaming output on platforms such as Skype and Zoom, the bar can be remarkably low, with stuttering, jerky video, and a whole range of potential compression artifacts, as well as ‘smoothing’ algorithms designed to reduce their effects – which in themselves constitute an additional range of ‘inauthentic’ effects that we have accepted as corollaries to the constraints and eccentricities of live streaming.