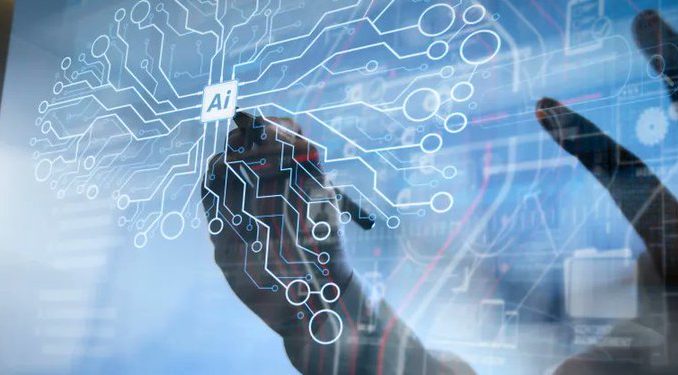
According to Gartner, artificial intelligence will create $2.9 trillion of business value and 6.2 billion hours of worker productivity globally in 2022. Many companies are aiming for an AI-first strategy where machine learning, in addition to optimizing business processes, is now also being used to rethink business strategies. An AI-first approach strategy makes AI the core of the company used to optimize forecasting, customer support, marketing, product, manufacturing, fault detection, and to learn about customer preferences and innovative ways to create competitive advantage.
In the ideal world, AI would be assisting with every phase of decision making fully embedded into systems and fully transparent to employees and customers. But to achieve this goal, fundamental changes need to take place including a top-down shift in mindset, as well as implementation of MLOps tools to help IT teams to overcome technical hurdles that can prevent AI from reaching its full potential.
Here are five steps a company can take to shift to an AI-first strategy.
1. Make AI the center of the business – Successful adoption of AI relies on strong executive-leadership support. Cross functional teams are put in place where subject matter experts such as radiologists or insurance specialists are on board, in addition to technical contributors. A full range of professionals that have touchpoints with AI projects including business users, software engineers, data scientists, data analysts, testers, architects, and product managers need to be involved so organizations can leverage AI faster and machine learning models can move into production more smoothly.
2. Make AI work in the long run – Models are typically designed by data scientists in ideal conditions. Moving from research to production is difficult, and many times this is the first barrier. If this is successful, then we have the maintenance challenge Many AI projects hit a wall due to a lack of clean and reliable data, direct access to different types of data storage, and a shortage of computing resources. When data scientists are building models, they should work with DevOps teams to develop a longer-term plan for data management, deployment, and AI system monitoring to ensure a successful implementation and smooth ongoing maintenance and operations.
3. Minimize tedious data preparation tasks – The tremendous task of data preparation, integration, and model training required so AI systems can go into production burns up a tremendous amount of time for the most scare resource, trained data scientists. Many of these tasks can be automated to streamline data pipelines, more easily move workloads from research to production, detect a degradation in performance, or detect a drift in results indicating that the models need to be retrained with more complete or fresher data.
4. Leverage off-the-shelf AI – Previously, only big players like Google and Facebook had the deep pockets to make AI/ML models a reality with access to the needed technology and hard to find data scientists. Today many companies are providing cutting edge open-source frameworks, tools, libraries, and models for research exploration and large-scale production deployment. The companies that jump ahead are the ones that can quickly utilize and customize open-source solutions for computer vision, language processing, speech recognition, and other commonly used functions.
5. Implement a best price-performance strategy -Today’s infrastructure landscape is a jungle. There are endless combinations of compute options that a data scientist can utilize for different AI workloads including CPUs, GPUs, AI accelerators, cloud, on–prem, hybrid cloud, co-location, and others. As a result, there is a lot of complexity, and unforeseen challenges executing jobs to achieve high performance at a reasonable price. Understanding the budgetary impact of AI and choosing the most cost-effective infrastructure can lower the overall cost of AI, accelerate innovation, limit risk, and speed up time to market.
With the new online economy, in 2022 companies will race to use AI insights to become more competitive by being truly data driven. As difficult as this past year was, it has proven that data analytics is the key to better business decisions and recent innovations have accelerated the process of companies re-inventing themselves to digitally transform their businesses.
By making AI central to the entire IT organization enterprises can become a step closer to leveraging AI as a strategic resource to reap the full benefits both in the short and for years to come.
About the author: Orly Amsalem is the vice president of AI innovation and business development at cnvrg.io, where she works to promote the company’s data-driven strategy and AI-driven tools for software developers, citizen data-scientists, and business users. She has many years of experience in both business and technical roles. She served as a principal business development manager and a senior product manager for AI/ML based anti-piracy applications at Synamedia. At Cisco she held positions as a senior product manager for AL/ML products and the lead for big data and data science teams, and at Coorelor Technologies she worked as a data analytics and BI manager.