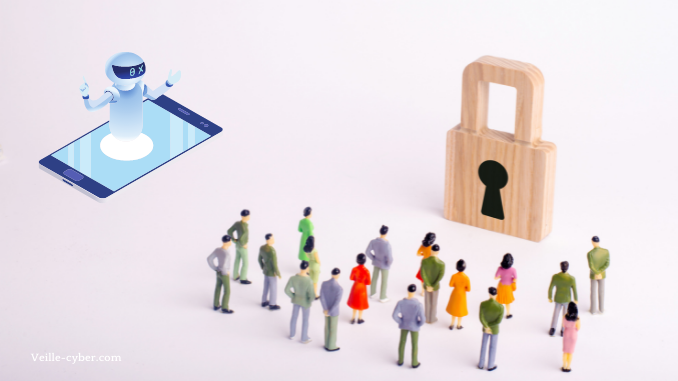
The cybersecurity industry is rapidly embracing the notion of “zero trust”, where architectures, policies, and processes are guided by the principle that no one and nothing should be trusted.
However, in the same breath, the cybersecurity industry is incorporating a growing number of AI-driven security solutions that rely on some type of trusted “ground truth” as reference point.
How can these two seemingly diametrically opposing philosophies coexist?
This is not a hypothetical discussion. Organizations are introducing AI models into their security practices that impact almost every aspect of their business, and one of the most urgent questions remains whether regulators, compliance officers, security professionals, and employees will be able to trust these security models at all.
Because AI models are sophisticated, obscure, automated, and oftentimes evolving, it is difficult to establish trust in an AI-dominant environment. Yet without trust and accountability, some of these models might be considered risk-prohibitive and so could eventually be under-utilized, marginalized, or banned altogether.
One of the main stumbling blocks associated with AI trustworthiness revolves around data, and more specifically, ensuring data quality and integrity. Afterall, AI models are only as good as the data they consume.
And yet, these obstacles haven’t discouraged cyber security vendors, which have shown unwavering zeal to base their solutions on AI models. By doing so, vendors are taking a leap of faith, assuming that the datasets (whether public or proprietary) their models are ingesting adequately represent the real-life scenarios that these models will encounter in the future.
The data used to power AI-based cybersecurity systems faces a number of further problems: