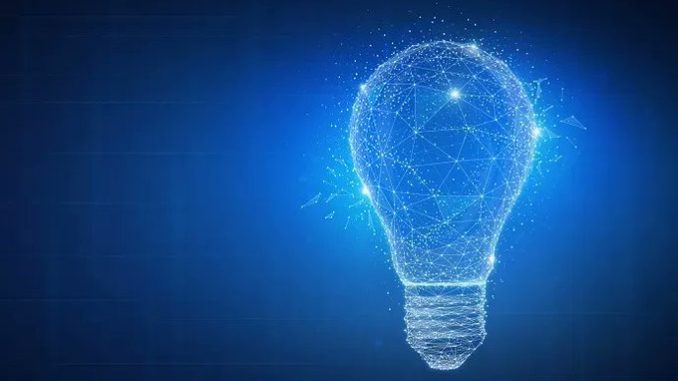
Researchers found that machine learning can play an important role in hip fracture classification, with algorithms performing better than human clinicians in some cases.
– A new machine-learning method used to identify and classify hip fractures has demonstrated its ability to outperform human clinicians.
Two convolutional neural networks (CNNs) developed at the University of Bath identified and classified hip fractures from X-rays with a 19 percent greater degree of accuracy and confidence than hospital-based clinicians.
The researchers from Bath’s Centre for Therapeutic Innovation and Institute for Mathematical Innovation and their colleagues from the Royal United Hospitals Trust Bath, North Bristol NHS Trust, and Bristol Medical School, set out to create a new process to assist clinicians in making hip fracture care more efficient.
The team used a total of 3,659 hip X-rays, classified by at least two experts, to train and test the machine-learning neural networks, which achieved an overall accuracy of 92 percent.
Hip fractures are a significant cause of morbidity and mortality in the elderly, creating high health costs and challenges to social care. Classifying a fracture before surgery is critical to assist surgeons in selecting the right interventions to treat the fracture, restore mobility, and improve patient outcomes.
The ability to accurately and quickly classify a fracture is critical. According to the press release, delays to surgery greater than 48 hours can increase the risk of adverse outcomes and mortality.
Depending on the part of the joint it occurs in, fractures are divided into three classes: intracapsular, trochanteric, or subtrochanteric. Some treatments determined by the fracture classification can cost up to 4.5 times as much as others.