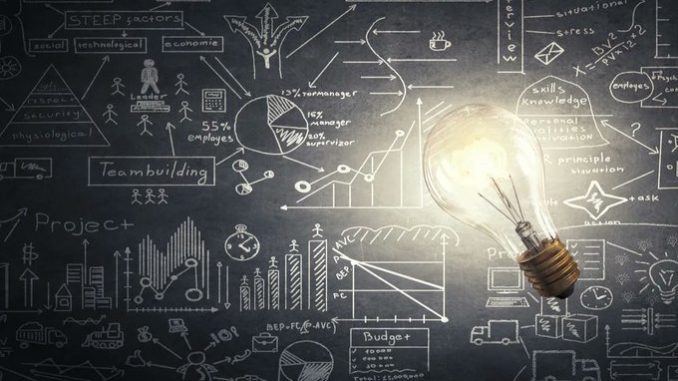
Last year, MIT developed an AI/ML algorithm capable of learning and adapting to new information while on the job, not just during its initial training phase. These “liquid” neural networks (in the Bruce Lee sense) literally play 4D chess — their models requiring time-series data to operate — which makes them ideal for use in time-sensitive tasks like pacemaker monitoring, weather forecasting, investment forecasting, or autonomous vehicle navigation. But, the problem is that data throughput has become a bottleneck, and scaling these systems has become prohibitively expensive, computationally speaking.
On Tuesday, MIT researchers announced that they have devised a solution to that restriction, not by widening the data pipeline but by solving a differential equation that has stumped mathematicians since 1907. Specifically, the team solved, “the differential equation behind the interaction of two neurons through synapses… to unlock a new type of fast and efficient artificial intelligence algorithms.”
“The new machine learning models we call ‘CfC’s’ [closed-form Continuous-time] replace the differential equation defining the computation of the neuron with a closed form approximation, preserving the beautiful properties of liquid networks without the need for numerical integration,” MIT professor and CSAIL Director Daniela Rus said in a Tuesday press statement. “CfC models are causal, compact, explainable, and efficient to train and predict. They open the way to trustworthy machine learning for safety-critical applications.”