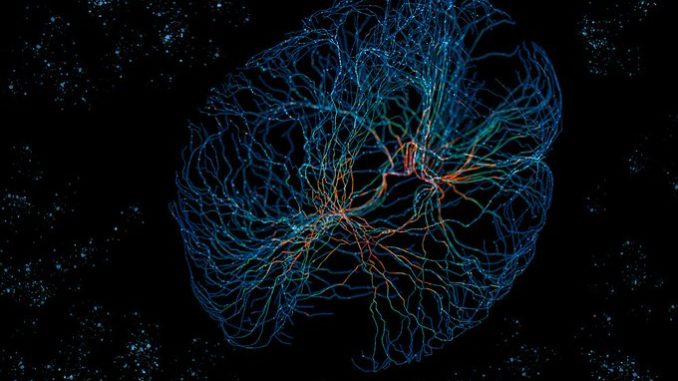
Computing systems that appear to generate brain-like activity may be the result of researchers guiding them to a specific outcome.
Neural networks, a type of computing system loosely modeled on the organization of the human brain, form the basis of many artificial intelligence systems for applications such speech recognition, computer vision, and medical image analysis.
In the field of neuroscience, researchers often use neural networks to try to model the same kind of tasks that the brain performs, in hopes that the models could suggest new hypotheses regarding how the brain itself performs those tasks. However, a group of researchers at MIT is urging that more caution should be taken when interpreting these models.
In an analysis of more than 11,000 neural networks that were trained to simulate the function of grid cells — key components of the brain’s navigation system — the researchers found that neural networks only produced grid-cell-like activity when they were given very specific constraints that are not found in biological systems.
“What this suggests is that in order to obtain a result with grid cells, the researchers training the models needed to bake in those results with specific, biologically implausible implementation choices,” says Rylan Schaeffer, a former senior research associate at MIT.
Without those constraints, the MIT team found that very few neural networks generated grid-cell-like activity, suggesting that these models do not necessarily generate useful predictions of how the brain works.