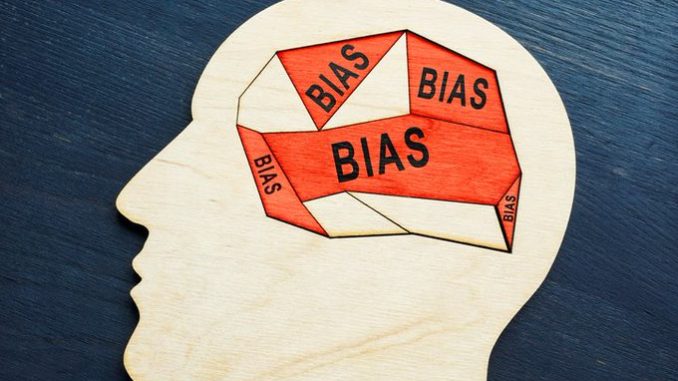
Bias gives AI a bad reputation, and there are good reasons for that. With the rising use of AI to recommend products, screen resumes, rate credit risk scoring, and more, bias in AI will impact our businesses and lives.
“Biases within AI tools are potentially dangerous for Asia — but biases about AI’s use in Asia could be even more so,” stated MIT Technology Review in its report Asia’s AI agenda The ethics of AI.
The report surveyed 871 senior business leaders in 13 economies within Asia. These participants in the AI ecosystem are aware of the embedded biases — race, gender, or socioeconomic status — within AI tools. They are also concerned with the harm AI causes through facilitating over-policing of minority communities or economic exclusion.
The black box mystery
The concern of AI bias has also escalated with the rising use of “black box” models, where AI algorithms are developed using deep neural networks, making it very difficult for data scientists and developers to explain how they work mathematically.
“Some legal scholars argue that AI codes are ambiguous and lack accountability,” stated the MIT Technology Review’s report.
The mistrust intensifies when “black box” models are combined with automation, where businesses respond and behave robotically based on the results of the AI models.
“While Asian decision-makers are concerned about a potentially negative impact, particularly where jobs are concerned, optimism is the more dominant sentiment, which will propel the use of AI in Asia,” stated the report.
Salvaging trust in AI
With the increasing use of AI in Asia, MIT Technology Review’s report stated that most business leaders (55%) of AI should be government-regulated. Governments in the region are also stepping up to build trust in AI.
Last month, the Monetary Authority of Singapore (MAS) released white papers that detail assessment methodologies to guide financial institutions for responsible use of AI based on fairness, ethics, accountability, and transparency principles. The Australian government also published the country’s AI Ethics Framework as guidelines for businesses and governments to design, develop and implement AI responsibly. Governments in India, Malaysia, and China have also set up agencies to develop similar guidelines.
The rising effort to build trust in AI is expected to double the market size of responsible AI solutions in 2022, according to Forrester’s Predictions 2022. Gartner also predicted by 2024, 60% of AI providers will include a means to mitigate possible harm as part of their technologies.
AI bias could be desirable
Ethical AI is high on the agenda. But AI bias should not be mistaken with irresponsible or unethical AI, noted Svetlana Sicular, vice president analyst, Gartner, as all businesses run with some forms of biases.
“Some forms of bias are desirable — for instance, avoiding bad language and favoring empathic, polite, and patient language are forms of bias toward what you rightly think is important for conversations between AI-enabled systems and people,” she noted in a recent article.
AI bias is often the result of a combination of biases in the real world, data, algorithms, and business. Gartner’s group vice president Anthony Bradley called it the four stages of ethical AI.
He noted the actual bias in the real world is reflected in data bias. When the algorithm is built from this data set, the models will create output that exposes the data bias. When businesses act blindly upon the algorithmic bias, they will impact and reinforce the real-world bias, causing AI bias to be unethical.
AI bias is simply a reflection of the bias in the real world. Bradley noted organizations should actively look for the hidden unethical biases in the data and make ethical business decisions from the AI algorithms.
“Bias is a natural effect of learning,” added Sicular. “It cannot be completely eliminated, but it can be managed.”
Ethical AI = transparent AI + ethical business
“Mathematically speaking, ethical AI is the sum of transparent AI and ethical business bias,” noted Bradley.
To manage bias and achieve transparent AI, Gartner suggested using tools to remove or mitigate measurable harm and bias and build explainable AI models.
The research firm’s report on Innovation insights for bias detection/mitigation, explainable AI, and interpretable AI stated data scientists and developers are encouraged to use bias detection and mitigation tools when selecting the data and training the AI model to compensate for bias in the data.
For AI to be explainable, data scientists and leaders should be able to describe the elements that facilitate the creation of the AI model, including algorithm uses, parameters of the models, and weightings of the parameters that are influential to the output.
While not all AI models are explainable, particularly among the “black box” models, they must be interpretable. This is part of running an ethical business and building a trustworthy AI.
Gartner suggested that models that have a significant impact on individuals, data professionals, and business leaders must be able to interpret and facilitate an understandable description of how and why the model is used for stakeholders or regulators.
“As has been true for eons, business bias remains the key to ethical behavior (AI is just the current flavor). Businesses should actively use AI to discover both ethical and unethical bias and act responsibly upon those findings,” Bradley concluded.