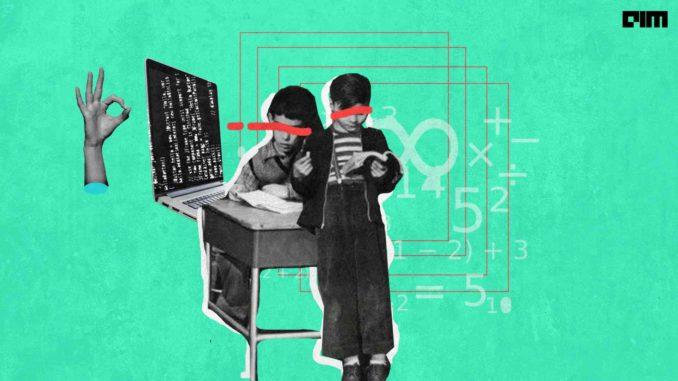
Neural networks achieve perfect generalisation, well past the point of overfitting, in some cases through grokking a pattern in data. In a potential ground breaking study, researchers from OpenAI (Alethea Power, Yuri Burda, Harri Edwards, Igor Babuschkin, Vedant Misra) have explored generalisation of neural networks on small algorithmically generated datasets. The team explored generalisation as a function of dataset size and discovered that smaller datasets call for large amounts of optimisation for generalisation.
The generalisation of overparameterised neural networks has long piqued the curiosity of the machine learning community as it runs counter to the intuitions drawn from classical learning theory. The researchers demonstrated that training networks on small algorithmically generated datasets are inclined to manifest unusual generalisation patterns–detached from performance on the training set–more conspicuously compared to datasets derived from natural data. The experiments can be reproduced on a single GPU.
A Cartel of Influential Datasets Is Dominating Machine Learning Research, New Study Suggests