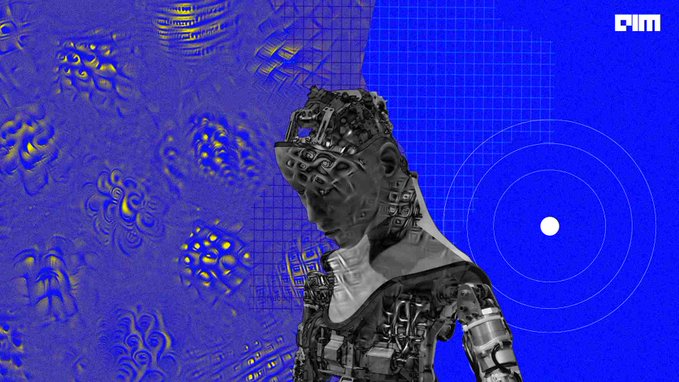
NeuPL is an efficient general framework that learns and represents policies in symmetric zero-sum games within a single conditional network.
The need for diverse policies for strategy games like StarCraft and poker are addressed by growing a robust policy population by iteratively training new policies against the existing ones. However, the approach has two challenges: Firstly, under a limited budget, the best response operators need truncating, resulting in under-trained good responses. Secondly, repeated learning of basic skills is wasteful and becomes intractable against stronger opponents.
Now, DeepMind and University College London have developed Neural Population Learning (NeuPL) to solve both issues. The researchers discovered that NeuPL guarantees the best responses under mild assumptions by showcasing a single conditional model policy. Moreover, NeuPL helps in transfer learning across policies. The research showed NeuPL can improve performance across various test domains. Additionally, it helps us understand how novel strategies are more accessible when the neural population increases.
RTS and NeuPL
Classical game theory is crucial to learning population strategies. The study uses “rock-paper-scissors” as an example where two strategies (rock, paper) is obtainable. Meanwhile, a distinct population (scissors) can be defeated when both are set in opposition or revealed. It is also shown in Policy Space Response Oracle (PSRO). New policies are trained to respond to an amalgamation of policies with the help of a meta-strategy solver. Finally, a PSRO variation was used to overpower StarCraft in 2019.
In turn-based games, improving strategies means winning or losing. However, performance can sometimes be consequential when a population of pure strategies works against a single population. For example, picking strategies first can always beat a player going second in the meta-game.