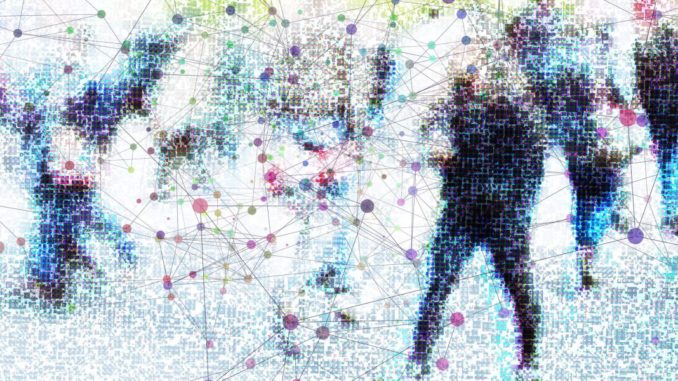
A recent Pew Research study found that a majority of experts and advocates worry AI will continue to focus on optimizing profits and social control and will not likely develop an ethical basis within the next decade. And in an academic study earlier this year, researchers from Cornell and the University of Pennsylvania found that two thirds of the machine learning researchers indicated AI safety should be prioritized more than it is presently. They also found that people are willing to place trust in AI when it is supported by existing international bodies such as the UN or the EU.
Some of these worries are based on early AI models that showed unintended biases. For example, Twitter’s algorithm for selectively cropping image previews showed an apparent bias for certain groups (Twitter later independently evaluated the algorithm and decided to take it down). Similar biases have been found not just in computer vision, but virtually all domains of machine learning.
We have seen several recent attempts to mitigate such problems. Last year, for example, the Department of Defense published five AI principles, recommending that AI technology should be responsible, equitable, traceable, reliable and governable. Google, Zendesk, and Microsoft, also issued guidelines, offering a framework to reach ambitious goals around ethical AI development. These are all good places to start.
Ethical AI is still in its nascency but is becoming increasingly more important for companies to take action on. My team approached ethical AI from a first principles perspective and augmented it with research from other players. We came up with these principles as we develop our own ethical AI framework and hope they are helpful to other teams:
1. Articulate the problem you’re trying to solve and identify the potential for bias
The first step to developing ethical AI is clearly articulating the problem you are trying to solve. If you are developing a credit scoring algorithm, for example, outline exactly what it is you’d like your algorithm to determine about an applicant and highlight any data points that may unintentionally lead to bias (e.g., racial confounders based on where someone lives). This also means understanding any implicit biases engineers or product managers may have and ensuring these biases don’t get enshrined into the code. One way to identify biases at the design stage is to involve team members from the very start who have diverse perspectives, both in terms of their business functions (such as legal, product, and marketing) and in terms of their own experiences and backgrounds.
2. Understand your underlying datasets and models
Once you’ve articulated the problem and identified potentials for bias, you should study the bias quantitatively by instilling processes to measure diversity in your datasets and model performance across groups of interest. This means sampling training data to ensure it fairly represents groups of interest, and segmenting model performance by these groups of interest to ensure you don’t see degraded performance for certain groups. For example, when developing computer vision models, like sentiment detection algorithms, ask yourself: Do they work equally well for both men and women? For various skin tones and ages? It is critical to understand the makeup of your dataset and any biases that may be inadvertently introduced either in training or in production.
3. Be transparent and approachable
AI teams should also seek to better understand their AI models and transparently share that understanding with the right stakeholders. This could have several dimensions but should focus primarily on what your AI models can and can’t do and on the underlying dataset they were built on. Consider a content recommender system: Can you articulate how much information it needs before being able to surface relevant recommendations to your customers? What steps, if any, does it take to mitigate amplification of viewpoints and homogenization of the user experience? The more you understand the underlying AI technologies you are building, the better you can transparently explain them to your users and other teams internally. Google has provided a good example of this with model cards — simple explanations of its AI models that describe when the models work best (and when they don’t).
Source : https://venturebeat.com/2021/08/29/how-to-make-ai-more-ethical/